Exploring AI Hardware Applications in Experiential Learning Environments
Project Members: 1Andrea Ramirez-Salgado, 2Tanvir Hossain, 2Tamzidul Hoque, PhD., 1Swarup Bhunia, PhD., 1Mary Jo Koroly, PhD., Bradford Davey, 1Pavlo “Pasha” Antonenko, PhD. 1University of Florida, 2University of Kansas
Background
-
A matter of national security for the US is supporting the development of the semiconductor and microchip workforce to address the chip shortage and its negative consequences.
-
Higher education institutions are establishing programs to train the necessary workforce and address the CHIPS and Science Act passed by US legislature in 2022.
-
Students pursuing engineering degrees are more inclined to
pursue software design rather than focus on hardware, primarily
due to the perceived complexities and the time-consuming nature of electronic manufacturing processes. -
We have undertaken a project funded by the NSF IUSE program to explore the usability and feasibility of AI-focused hardware activities specifically for first-year undergraduate students across various engineering disciplines.
-
We aim to demystify AI and expand conceptions of ML to cultivate situational interest in computer hardware design and support hardware engineering career choice.
Theoretical Framework
-
We developed a conceptual framework to promote career choice by providing hands-on experiential learning. Career choice is supported by interest development, self-efficacy and outcome expectations.
-
RQ: What are student’s perceptions and practices in an undegraduate AI hardware curriculum?
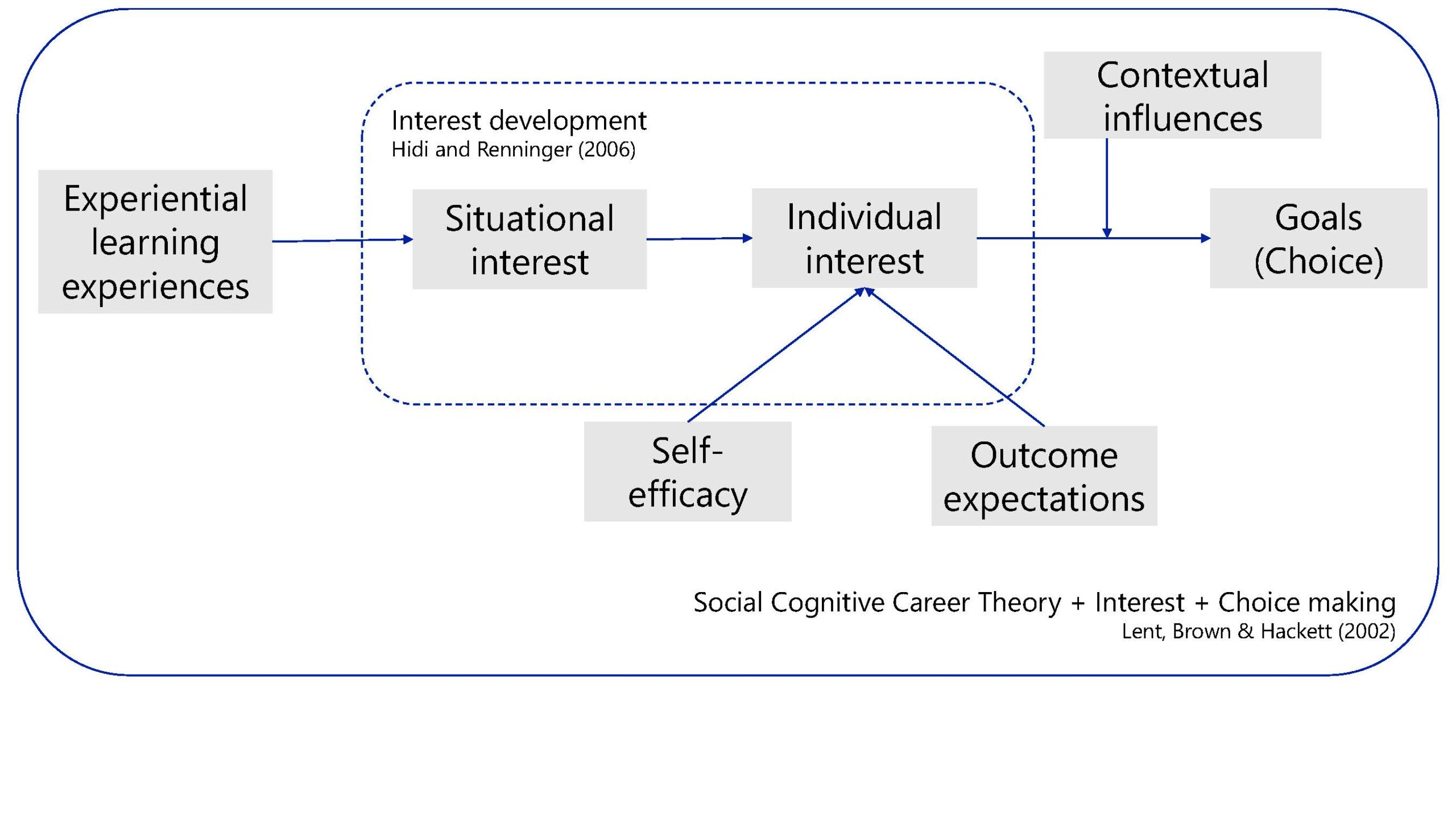
Implementation
-
n=22 first-year engineering students are participating in a first implementation of the AI hardware curriculum (6 girls and 16 boys).
-
The curriculum is integrated into an undergraduate course open to students in all engineering disciplines. No previous knowledge or skills are required.
-
We scaffold each activity by offering a hands-on explanation of the hardware device. Afterward, we encourage them to collect data and understand the results.
-
We provide the necessary codes and libraries for each activity.
-
By the end of the semester, students will collaborate in groups to develop a real-world application using the AI hardware approach.
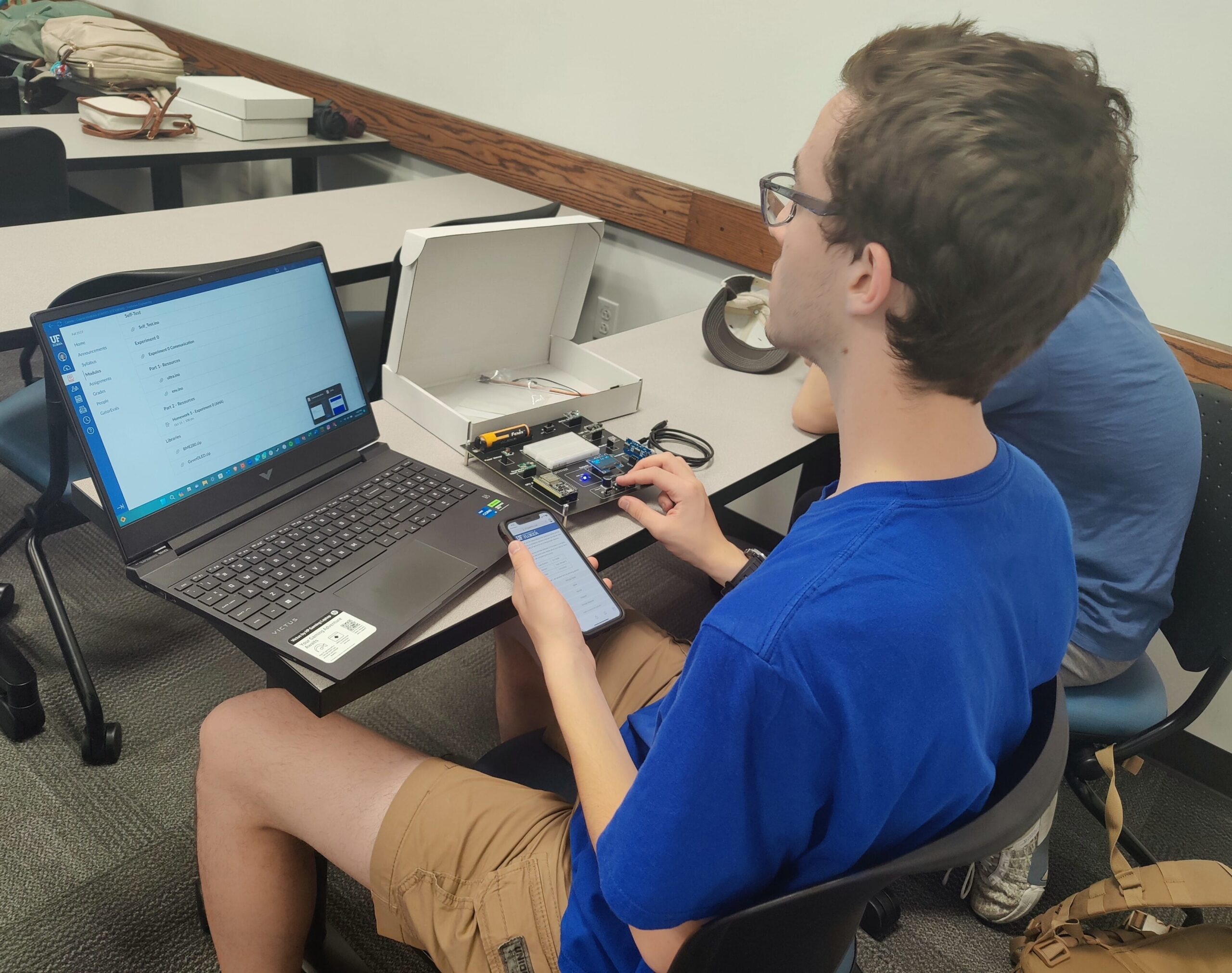
Artificial Intelligence IoT activities – Examples
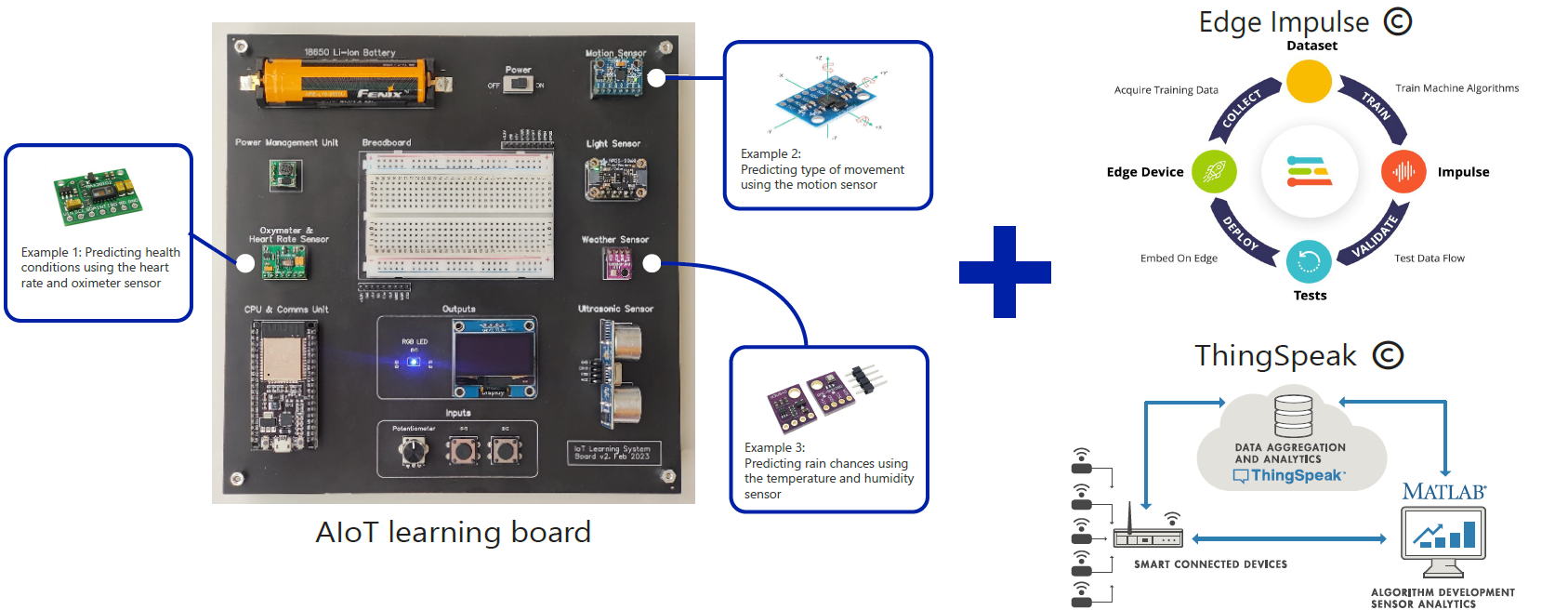
Preliminary Results
-
Students value the hands-on approach as it helps them quickly grasp AI hardware concepts.
-
They enjoy experimenting with the codes and find it both enjoyable and beneficial.
-
Our approach is seen by students as more meaningful compared to simulations or videos.
-
A suggested improvement is to include additional comments in the code to assist learners with limited or no experience in the programming language.
-
The majority of students have been able to successfully complete all activities.
Reference
Hidi, S., & Renninger, K. A. (2006). The four-phase model of interest development. Educational Psychologist, 41(2), 111–127.
Lent, R. W., Brown, S. D., & Hackett, G. (2002). Social cognitive career theory. Career choice and development, 4(1), 255-311.